
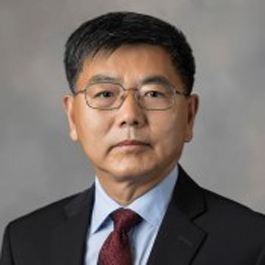
Advancing Omics Data Analysis Through the Integration of Imaging Physics and Deep Learning
Dr. Lei Xing
Jacob Haimson & Sarah S. Donaldson Professor
Director of Medical Physics Division Department of Radiation Oncology
Molecular Imaging Program at Stanford (MIPS)
Stanford University
Abstract: Maximizing the utilization of omics data for informed decisions has been a crucial objective in the realm of biomedicine. As we navigate this exciting era of AI, a vigorous debate has emerged concerning the superior approach for tabular omics data analysis: deep learning or decision trees. To definitively address this question, our study delved into the factors contributing to deep learning’s frequent inability to outperform decision trees in many scenarios. We found that, by appropriately reconfiguring the data, deep learning consistently outperforms both classical machine learning and other techniques. We showcase the superior performance of the approach across various omics datasets and demonstrate that our study marks an important advancement in the processing of omics data and have significant implications in the field of biomedical informatics.
Bio: Dr. Lei Xing is the Jacob Haimson & Sarah S. Donaldson Professor and Director of Medical Physics Division of Radiation Oncology Department at Stanford University School of Medicine. He also holds affiliate faculty positions in Department of Electrical engineering, Biomedical Informatics, Bio-X and Molecular Imaging Program at Stanford. Dr. Xing obtained his PhD in Physics from the Johns Hopkins University in 1992 and received his Medical Physics training at the University of Chicago. His research has been focused on AI in medicine, medical imaging, treatment planning, medical imaging, image guided interventions, and applications of molecular imaging in radiation oncology. He has made unique and significant contributions to each of the above areas. Dr. Xing is an author on more than 400 peer reviewed publications. He is an inventor/co-inventor on many issued and pending patents, and a co- investigator or principal investigator on numerous NIH, DOD, NSF, RSNA, AAPM, Komen, ACS and corporate grants. He is a fellow of AAPM, ASTRO and AIMBE.
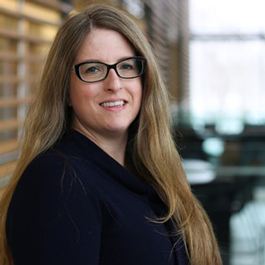
Radiomics, Radiogenomics, and Medical AI for Health Innovation
Dr. Amber Simpson
Canada Research Chair in Biomedical Computing and Informatics
Associate Professor, School of Computing
Director, Center for Health Innovation
Queen’s University, Canada
Abstract: How can we harness the power of artificial intelligence and machine learning to identify tailored treatments for patients with cancer? In this talk, we seek to transform how clinicians treat patients with cancer using a data-driven approach. By defining relevant biomarkers to guide targeted treatments, and ultimately improve human health, we will move the field from the “treat everyone to help a few” paradigm towards precision medicine. We will leverage state-of-the-art machine learning technologies for biomedical data integration and exploration. Specifically, to develop, validate, and translate to clinical practice two important field innovations. The first will develop benchmarking and novel machine learning-based predictive and prognostic imaging biomarkers to better select patients for optimal treatment. The second innovation will be to integrate computation modeling of multi-scale, multi-resolution biomedical data to elucidate new phenotypes, such as appearance, development, and behavior, of cancer tumors. Ultimately, through developing new computational strategies, we will advance biomedical data science and computer-aided surgery to improve human health.
Bio: Dr. Amber Simpson is a Canada Research Chair in Biomedical Computing and Informatics. Her research focuses on evaluating cancer patient prognosis, guiding treatment planning, understanding underlying patterns of disease, and assessing the impact and bias of computational models in medicine. Her research goal is to ensure cancer patients receive optimized and equitable care through the development of novel computational strategies. Her lab has individuals from a variety of academic backgrounds, including computer science, life science, public health, engineering, philosophy, medicine, and mathematics. Each person is passionate about the goal and can contribute a unique perspective on current healthcare issues, allowing us to improve and enrich our research. Her lab also collaborates frequently with industry partners and research institutions on a global scale. With their help, her research is able to push the boundaries of cancer research and move one step closer to the ultimate goal of finding a cure.